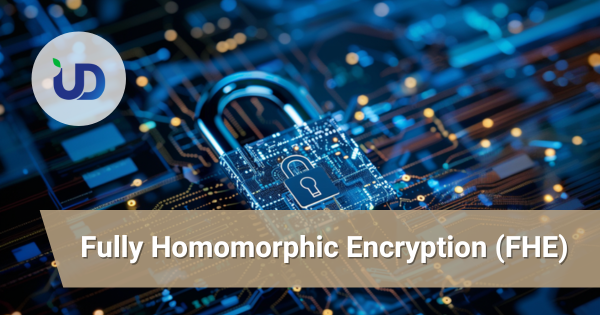
Understanding the Commercial Value of AI+FHE Homomorphic Encryption in One Article
As of October 13th, the data statistics for BTC, ETH, and TON on the TrendX platform are as follows:
The discussion volume of BTC last week was 12.52K, a decrease of 0.98% from the previous week, and the price last Sunday was $63,916, an increase of 1.62% from the previous Sunday;
The discussion volume of ETH last week was 3.63K, an increase of 3.45% from the previous week, and the price last Sunday was $2,530, a decrease of 4% from the previous Sunday;
The discussion volume of TON last week was 782, a decrease of 12.63% from the previous week, and the price last Sunday was $5.26, a decrease of 0.25% from the previous Sunday;
Homomorphic Encryption (Fully Homomorphic Encryption, FHE) is a highly promising technology in current cryptography, with its core feature allowing direct computation on encrypted data without the need for decryption, providing strong support for privacy protection and data processing. FHE can be widely applied in finance, healthcare, cloud computing, machine learning, voting systems, Internet of Things, and blockchain privacy protection. However, despite the broad prospects for FHE applications, it still faces challenges in its commercialization path.
Potential and Application Scenarios of FHE
The greatest advantage of homomorphic encryption lies in privacy protection. Imagine that Company A needs to utilize the computing power of Company B to analyze its data, but does not want Company B to access the specific content of this data. FHE can play a role in this scenario: Company A can encrypt the data, transmit it to Company B for computation, and the computation results remain encrypted, which can be decrypted by Company A to obtain the analysis results. In this way, data privacy is effectively protected, and Company B can complete the necessary computations.
This privacy protection mechanism is particularly important for data-sensitive industries such as finance and healthcare. In addition, with the development of cloud computing and artificial intelligence, data security has become an increasing focus. In these scenarios, FHE can provide multiparty computation protection, allowing all parties to collaborate without exposing sensitive information. Especially in blockchain technology, FHE enhances the transparency and security of data processing through on-chain privacy protection and privacy transaction review.
Comparison of FHE with Other Encryption Methods
In the Web3 domain, FHE, Zero-Knowledge Proofs (ZK), Secure Multiparty Computation (MPC), and Trusted Execution Environments (TEE) are the main privacy protection methods. Unlike ZK, FHE can perform various operations on encrypted data without the need to decrypt the data first. MPC allows parties to perform computations on encrypted data without sharing private information. TEE provides secure computation environments, but with relatively limited flexibility in data processing.
These encryption technologies have their own advantages, but FHE excels in supporting complex computational tasks. However, FHE still faces challenges in practical applications due to high computational costs and poor scalability, making it often inadequate for real-time applications.
Limitations and Challenges of FHE
Despite the strong theoretical foundation of FHE, it encounters practical challenges in commercial applications.
-
Large-scale computational costs: FHE requires a significant amount of computing resources, leading to a significant increase in computational costs compared to unencrypted computations. For high-degree polynomial operations, the processing time grows polynomially, making it difficult for FHE to meet real-time computation needs. To reduce costs, FHE relies on dedicated hardware acceleration, but this also increases deployment complexity.
-
Limited operational capabilities: Although FHE can perform addition and multiplication on encrypted data, its support for complex nonlinear operations is limited, posing a bottleneck for applications involving deep neural networks and other artificial intelligence applications. Current FHE schemes are mainly suitable for linear and simple polynomial computations, significantly restricting the application of nonlinear models.
-
Complexity of multi-user support: FHE performs well in single-user scenarios, but when dealing with multi-user datasets, system complexity sharply increases. The multi-key FHE framework proposed by Lopez-Alt et al. in 2013 allows operations on encrypted datasets with different keys, but it significantly increases the complexity of key management and system architecture.
Integration of FHE with Artificial Intelligence
In the current data-driven era, artificial intelligence (AI) is widely applied in various fields. However, due to concerns about data privacy, users are often unwilling to share sensitive data such as medical and financial information. FHE provides a privacy protection solution for the AI field. In cloud computing scenarios, data is usually encrypted during transmission and storage, but often in plaintext during processing. With FHE, users' data can be processed while remaining encrypted, ensuring data privacy.
This advantage is particularly important under regulations such as GDPR, as these regulations require users to be informed about data processing methods and ensure data protection during transmission. End-to-end encryption provided by FHE ensures compliance and data security.
Current Applications and Projects of FHE in Blockchain
The application of FHE in blockchain mainly focuses on protecting data privacy, including on-chain privacy, AI training data privacy, on-chain voting privacy, and on-chain privacy transaction review. Currently, many projects utilize FHE technology to advance privacy protection. For example, the FHE solution built by Zama based on TFHE technology is widely used in projects such as Fhenix, Privasea, IncoNetwork, and MindNetwork.
Zama: Based on TFHE technology, it focuses on Boolean operations and low-bit-length integer operations, and constructs an FHE development stack for blockchain and AI applications.
More information:
https://app.trendx.tech/project/Zama/df5cf223
Octra: Developed a new smart contract language and HyperghraphFHE library suitable for blockchain networks.
Privasea: Utilizes FHE to achieve privacy protection in AI computation networks, supporting various AI models.
More information:
https://app.trendx.tech/project/Privasea/998be4de
MindNetwork: Combines FHE with artificial intelligence to provide a decentralized and privacy-protected AI environment.
More information:
https://app.trendx.tech/project/Mind%20Network/45d76376
Fhenix: As an Ethereum Layer 2 solution, it supports FHE Rollups and FHE Coprocessors, is compatible with EVM, and supports smart contracts written in Solidity.
More information:
https://app.trendx.tech/project/Fhenix/3e8d2b3b
Research Data
Conclusion
As an advanced technology capable of performing computations on encrypted data, FHE has significant advantages in protecting data privacy. Although current commercial applications of FHE still face challenges such as high computational costs and poor scalability, these issues are expected to be gradually resolved through hardware acceleration and algorithm optimization. Furthermore, with the development of blockchain technology, FHE will play an increasingly important role in privacy protection and secure computation. In the future, FHE may become the core technology supporting privacy-protected computation, bringing new revolutionary breakthroughs in data security.
Follow TrendX
TrendX - No.1 Web3 investment opportunity platform, leveraging industry-leading AI analysis technology and on-chain and off-chain trend tracking technology to analyze real-time data from billions of sources, capture investment opportunities, and provide investment advice to users through intuitive interaction. Committed to becoming the preferred Web3 investment platform, adhering to the concept of 'change is opportunity'.
Website: https://app.trendx.tech/
Twitter: https://twitter.com/TrendX_tech
Investment carries risks, and the projects are for reference only. Please bear the risks on your own.