Decoding AI AGENT: The intelligent power to shape the future new economic ecology
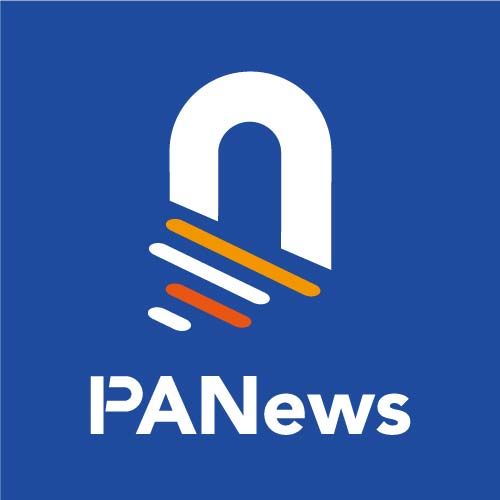
Reprinted from panewslab
02/08/2025·5D1. Background Overview
1.1 Introduction: The "new partner" in the intelligent era
Every cryptocurrency cycle brings new infrastructure that drives the entire industry.
- In 2017, the rise of smart contracts gave birth to the booming ICO.
- In 2020, DEX's mobile pool brought about a summer boom in DeFi.
- In 2021, the release of a large number of NFT series marks the arrival of the era of digital collectibles.
- In 2024, pump.fun's outstanding performance led the craze for memecoin and launch platforms.
It should be emphasized that the start of these vertical fields is not only due to technological innovation, but also the result of the perfect combination of financing models and bull market cycles. When the opportunity meets the right time, it can lead to huge changes. Looking ahead to 2025, it is clear that the emerging field of the 2025 cycle will be AI agents. This trend peaked last October with the $GOAT token launched on October 11, 2024 and a market capitalization of $150 million on October 15. Immediately afterwards, Virtuals Protocol launched Luna, which debuted with the IP live broadcast image of Girl Next Door, igniting the entire industry.
So, what exactly is an AI Agent?
Everyone must be familiar with the classic movie "Resident Evil", which is an AI system called Queen Heart. Queen of Heart is a powerful AI system that controls complex facilities and security systems that can automatically perceive the environment, analyze data, and act quickly.
In fact, AI Agent has many similarities to the core functions of Queen Hearts. Reality AI Agents play a similar role to some extent, they are the "guardians of wisdom" in the modern technology field, helping businesses and individuals to deal with complex tasks through autonomous perception, analysis and execution. From autonomous vehicles to intelligent customer service, AI Agent has penetrated into all walks of life and has become a key force in improving efficiency and innovation. These autonomous agents, like invisible team members, have the full range of capabilities from environmental perception to decision-making execution, gradually penetrate into various industries, promoting the dual improvement of efficiency and innovation.
For example, an AI AGENT can be used to automate transactions, manage portfolios and execute transactions in real time based on data collected from Dexscreener or social platform X, and continuously optimize its performance in iterations. AI AGENT is not a single form, but is divided into different categories according to specific needs in the crypto ecosystem:
1. Execution AI Agent: Focus on completing specific tasks such as trading, portfolio management or arbitrage, aiming to improve operational accuracy and reduce the time required.
2. Creative AI Agent: used for content generation, including text, design and even music creation.
3. Social AI Agent: As an opinion leader on social media, interact with users, build communities and participate in marketing activities.
4. Coordinated AI Agent: Coordinate complex interactions between systems or participants, especially suitable for multi-chain integration.
In this report, we will explore the origin, current status and broad application prospects of AI Agents, analyze how they reshape the industry landscape, and look forward to their future development trends.
1.1.1 Development history
The development history of AI AGENT demonstrates the evolution of AI from basic research to widespread application. At the Dartmouth Conference in 1956, the term “AI” was first proposed, laying the foundation for AI as an independent field. During this period, AI research focused mainly on symbolic methods, giving birth to the first AI programs, such as ELIZA (a chatbot) and Dendral (an expert system in the field of organic chemistry). This stage also witnessed the first proposal of neural networks and the initial exploration of the concept of machine learning. However, AI research during this period was severely restricted by the computing power limitations at that time. Researchers have encountered great difficulties in natural language processing and algorithm development that mimics human cognitive functions. In addition, in 1972, mathematician James Lightthill submitted a report published in 1973 on the status of ongoing AI research in the UK. The Lightthill report basically expresses the overall pessimism about AI research after the early excitement period, triggering a huge loss of confidence in AI by British academic institutions (including funding agencies). After 1973, AI research funding decreased significantly, and the AI field experienced the first "AI winter", and doubts about the potential of AI increased.
In the 1980s, the development and commercialization of expert systems led global enterprises to adopt AI technology. This period has made significant progress in machine learning, neural networks and natural language processing, driving the emergence of more complex AI applications. The introduction of autonomous vehicles for the first time and the deployment of AI in various industries such as finance and medical care also mark the expansion of AI technology. But between the late 1980s and early 1990s, the AI field experienced a second "AI winter" as the market demand for dedicated AI hardware collapsed. In addition, how to scale up the AI system and successfully integrate it into practical applications remains an ongoing challenge. But at the same time, in 1997, IBM's Deep Blue Computer defeated world chess champion Gary Kasparov, a milestone in AI's ability to solve complex problems. The revival of neural networks and deep learning laid the foundation for AI development in the late 1990s, making AI an integral part of the technological landscape and beginning to influence everyday life.
By the beginning of this century, advances in computing power have driven the rise of deep learning, and virtual assistants such as Siri demonstrated the practicality of AI in consumer applications. In the 2010s, further breakthroughs were made in generative models such as reinforcement learning agents and GPT-2, pushing conversational AI to new heights. In this process, the emergence of the Large Language Model (LLM) has become an important milestone in the development of AI, especially the release of GPT-4, which is regarded as a turning point in the field of AI agents. Since OpenAI released the GPT series, large-scale pre-trained models have demonstrated language generation and understanding capabilities beyond traditional models through tens of billions or even hundreds of billions of parameters. Their outstanding performance in natural language processing allows AI agents to demonstrate logically clear and organized interaction capabilities through language generation. This enables AI agents to be applied to chat assistants, virtual customer service and other scenarios, and gradually expand to more complex tasks (such as business analysis, creative writing).
The learning ability of large language models provides higher autonomy for AI agents. Through Reinforcement Learning technology, AI agents can continuously optimize their own behavior and adapt to dynamic environments. For example, in AI-driven platforms such as Digimon Engine, AI agents can adjust behavioral strategies based on player inputs to truly achieve dynamic interaction.
From early rules systems to large language models represented by GPT-4, the history of the development of AI agents is an evolutionary history that constantly breaks through technological boundaries. The emergence of GPT-4 is undoubtedly a major turning point in this process. With the further development of technology, AI agents will be more intelligent, scenario-based and diversified. The large language model not only injects "wisdom" soul into AI agents, but also provides it with the ability to collaborate across fields. In the future, innovative project platforms will continue to emerge, continuing to promote the implementation and development of AI agent technology and lead a new era of AI-driven experience.
1.2 Working principle
What makes AIAGENT different from traditional robots is that they are able to learn and adapt over time, making meticulous decisions to achieve their goals. They can be seen as technologically advanced, evolving players in the crypto field, able to act independently in the digital economy.
The core of AI AGENT lies in its "intelligence" - that is, through algorithms, simulate the intelligent behavior of humans or other organisms to automatically solve complex problems. The workflow of AI AGENT usually follows the following steps: perception, reasoning, action, learning, and adjustment.
1.2.1 Perception module
AI AGENT interacts with the outside world through perception modules to collect environmental information. This part of the function is similar to the human senses, using sensors, cameras, microphones and other devices to capture external data, which includes extracting meaningful features, identifying objects, or determining relevant entities in the environment. The core task of a perception module is to convert raw data into meaningful information, which usually involves the following techniques:
- Computer Vision: Used to process and understand image and video data.
- Natural Language Processing (NLP): Helps AI AGENT understand and generate human language.
- Sensor Fusion: Integrate data from multiple sensors into a unified view.
1.2.2 Reasoning and Decision Map
After sensing the environment, AI AGENT needs to make decisions based on the data. The reasoning and decision-making module is the "brain" of the entire system, which performs logical reasoning and strategy formulation based on the collected information. Use large language models, etc. as orchestrators or reasoning engines to understand tasks, generate solutions, and coordinate specialized models for specific functions such as content creation, visual processing, or recommendation systems.
This module usually uses the following techniques:
- Rule Engine: Make simple decisions based on preset rules.
- Machine learning models: including decision trees, neural networks, etc., used for complex pattern recognition and prediction.
- Reinforcement learning: Let AI AGENT continuously optimize decision-making strategies in trial and error to adapt to changing environments.
The reasoning process usually consists of several steps: first, the assessment of the environment, second, the calculation of multiple possible action plans based on the goals, and finally the selection of the optimal plan to execute.
1.2.3 Execution module
The execution module is the "hands and feet" of AI AGENT, which puts the decisions of the reasoning module into action. This part interacts with external systems or devices to complete the specified tasks. This may involve physical operations (such as robotic actions) or digital operations (such as data processing). The execution module depends on:
- Robot control system: used for physical operations, such as the movement of robotic arms.
- API call: Interact with external software systems, such as database queries or network service access.
- Automated process management: Perform repetitive tasks through RPA (Robot Process Automation) in an enterprise environment.
1.2.4 Learning module
The learning module is the core competitiveness of AI AGENT, which enables agents to become smarter over time. Through continuous improvements in feedback loops or “data flywheels”, the data generated in the interaction is fed back into the system to enhance the model. This ability to adapt and become more effective over time provides a powerful tool for businesses to improve decision-making and operational efficiency.
The learning module is usually improved by:
- Supervised learning: Use labeled data for model training, so that AI AGENT can complete tasks more accurately.
- Unsupervised learning: Discover potential patterns from unlabeled data to help agents adapt to new environments.
- Continuous learning: Keep the agent performing in a dynamic environment by updating the model in real-time data.
1.2.5 Real-time feedback and adjustment
AI AGENT optimizes its performance through continuous feedback loops. The results of each action are recorded and used to adjust future decisions. This closed-loop system ensures the adaptability and flexibility of AI AGENT.
1.3 Market status
1.3.1 Industry status
AI AGENT is becoming the focus of the market, bringing change to multiple industries with its huge potential as a consumer interface and an autonomous economic actor. Just as the potential of L1 block space in the previous cycle is incalculable, AI AGENT also showed the same prospects in this cycle.
According to the latest report from Markets and Markets, the AI Agent market is expected to grow from US$5.1 billion in 2024 to US$47.1 billion in 2030, with an annual compound growth rate (CAGR) of up to 44.8%. This rapid growth reflects the AI Agent's penetration in various industries and the market demand brought by technological innovation.
Source: LangChain Blog, 2025/1/20
Large companies have also invested significantly in open source proxy frameworks. Microsoft's AutoGen, Phidata and LangGraph development activities are becoming increasingly active, which shows that AI AGENT has greater market potential outside the crypto field, and TAM is also expanding, and investors continue to pay more attention to it, and they are more willing to do so. This gives a premium multiple.
From the perspective of deploying public chains, Solana is the main
battlefield, and other public chains such as Base chains have huge potential.
From the perspective of market awareness (Mindshare), FARTCOIN and AIXBT are far ahead. The birth of Fartcoin and GOAT are both derived from the terminal of truths AI AGENT model. During the dialogue between the goat model and opus (artificial intelligence tool), it was mentioned that Musk Musk likes to fart sounds, so this AI The model proposes to issue a token called Fartcoin and designs a series of promotion methods and gameplay. Fartcoin was thus born on October 18, slightly later than GOAT (October 11), and achieved a brief valuation of more than $1 billion in December 2024. Although initially considered a humorous view of the digital currency space, its rapid rise has prompted investors and analysts to study its fundamentals, market performance and potential longevity. Judging from the hot topics of social media, Fartcoin has hit the hot spots of AI AGENT.
AIXBT, ranked second, is an AI Agent based on the Base chain launched by
Virtuals Protocol. But unlike traditional meme tokens, it not only has
entertainment nature, but also provides users with powerful market analysis
functions through AI Agent technology. AIXBT uses proprietary AI engines to
extract hot topics and discussion trends from social media (such as Twitter)
and KOL resources to provide investors with real-time insights into market
changes. As part of the Virtuals Protocol ecosystem, AIXBT undertakes the
mission of leading investors to understand market dynamics and analyze
potential opportunities. Its core goal is to provide users with reliable
information support through technology and token mechanisms, thereby
optimizing investment decisions.
Source: cookie.fun, 2025/1/20
From a technical perspective, AI Agent technology is developing towards multimodal interaction and high autonomous decision-making capabilities. In 2024, the introduction of cross-modal learning and generative pre-trained models such as GPT family models enables AI Agents to better understand and process multiple forms of data, such as text, images, and speech. These technological breakthroughs significantly improve Agent's understanding and decision-making efficiency, allowing it to make autonomous decisions in more complex and dynamic environments. According to McKinsey's analysis, AI Agent's multimodal capabilities and cross-domain collaboration are becoming a symbol of the era of intelligence. This allows AI Agent to not only provide support for a single task, but also provide comprehensive information analysis and dynamic optimization suggestions in complex decision-making
1.3.2 Reasons for combining AI Agent and token economic model
The combination of AI Agent and token economic model is not only an inevitable trend in technological development, but also a built-in internal driving mechanism for its ecosystem for efficient, transparent and sustainable development. Here are a few key reasons:
1. Build a more efficient incentive system
The operation and optimization of AI Agents relies on large amounts of data acquisition, training, and reasoning, and these processes require strong incentives to continue to operate. For example:
- Data acquisition incentives: The token economy can provide direct returns to data providers, inspiring individuals or businesses to contribute high-quality labeled data or real-time market data.
- Inference task allocation: Through the token reward mechanism, AI Agent can complete complex computing tasks competitively, thereby optimizing its inference efficiency and accuracy.
- Promote innovation and collaboration: Tokenized reward systems can attract more developers and users to participate, forming a positive feedback loop for technology and ecosystems.
- Case: Some blockchain-based AI platforms (such as Ocean Protocol) promote the prosperity of the data market through token rewards data sharing behavior.
2. Assetization of AI Agent itself
Through tokenization, AI Agent can not only be a tool, but also become a new asset and create long-term wealth effects.
- Tokenized Identity: The data, skills and execution capabilities of AI Agent can be evaluated and priced. By issuing corresponding tokens, users can use their functions on demand.
- Investment value: Token holders of AI Agent can share the dividends of their growth, such as value appreciation brought by the increase in the market share of agents and the optimization of reasoning efficiency.
- Liquidity enhancement: The existence of tokens provides circulating market value for AI Agent, giving it trading and investment attributes, attracting more capital to enter this field.
- Case: For example, SingularityNET supports AI service transactions through tokens (AGIX), allowing AI Agent to be assetized and achieve sustainable development.
3. Support interaction and transactions between AI Agents
In the future, AI Agent will no longer be an isolated individual, but will form a huge Internet network. In this network, a decentralized token economic model is the key to achieving efficient interaction and value exchange.
- Payment and Settlement: AI Agent can complete task payment and service settlement through cryptocurrency, reducing the intermediate links of traditional payment systems and improving transaction efficiency.
- Value allocation: Through smart contracts, the results of collaboration between AI Agents (such as the optimization benefits of joint learning models) can be automatically distributed according to agreed rules to ensure fairness.
- Decentralized Autonomous Organization (DAO) Governance: The behavior of AI Agent can be managed through token holders' voting to ensure that its operations are transparent and in line with ecological interests.
- Case: In a decentralized AI network, AI Agent can exchange resources through tokens (such as data storage and computing power leasing) to achieve a self-driven collaboration system.
4. Improve system transparency and security
The token economic model combines blockchain technology to provide an untampered record and a transparent operating mechanism for the operation of AI Agent.
- Traceability and audit: All transactions, reasoning and data usage behaviors can be recorded on the chain to ensure the credibility and auditability of the system.
- Data security and privacy: Through token-incentive privacy computing, users can contribute data without leaking sensitive data, further enhancing security.
- Prevent abuse and cheating: The token model can set economic penalties for malicious behavior and reduce the possibility of bad behavior.
5. Accelerate the formation of a global, borderless AI economic ecosystem
The token economic model can break through geographical restrictions and allow global users to participate in the construction and use of AI Agents.
- Lower the entry threshold: The global circulation characteristics of cryptocurrencies can provide financial support to users or institutions without bank accounts, allowing more people to share the development dividends of AI.
- Global collaboration: Whether it is data sharing, AI training, or cross-border transactions, the token system provides the infrastructure for global collaboration and eliminates barriers to traditional economic systems.
- Ecological self-circulation: Through the token economy, the benefits of AI Agent can be directly fed back to development and ecological construction, achieving long-term development.
Overall, the combination of AI Agent and token economic model is not only a matching of technology and economic logic, but also an innovative form for the future digital economy. By introducing a token system, AI Agent can incentivize more efficient data and resources utilization, asset its own value, support interaction and transactions, improve transparency and security, and even build a global open economic ecosystem. This model is expected to become an important direction to promote the integration of AI and blockchain, laying the foundation for the further intelligence of the digital society.
2. AI Agent application analysis in crypto
2.1AI AGENT LAUNCHPAD
AI Agent Launchpad refers to a platform focusing on the issuance of smart agents and their related tokens, and its functions are similar to Meme coin issuance platforms such as Pump.fun. This platform enables users to easily create and deploy AI AGENTs and seamlessly integrate with social media platforms such as Twitter, Telegram, Discord, etc. to enable automated user interaction. This method greatly lowers the threshold for distribution and promotion, provides users with a more convenient creation experience, and expands the application areas of AI AGENT and promotes its application in a wider range of social and economic scenarios.
2.1.1Virtuals Protocol
In the emerging field of AI Agent Launchpad, we have to mention Virtuals Protocol. Virtuals Protocol is launched on Base. Users can easily deploy their own AI AGENT using VIRTUAL tokens.
- Creation and deployment: Each agent requires 100 VIRTUAL tokens to start, and the initial liquidity is ensured through the binding curve mechanism.
- Capitalization mechanism: After reaching a specific capitalization threshold, the agent enters a new stage, automatically deploys the liquidity pool, and smart contracts run independently.
- Autonomous interaction: Agents are able to automate transactions and other tasks and participate in community activities.
The Virtuals Protocol team demonstrates outstanding adaptability and strategic vision, and their path to success stems from a range of key transformation and innovative initiatives. The story begins at the end of 2021, when a group of young people from well-known companies such as Boston Consulting (BCG), Meta, etc. captured the opportunity of the GameFi boom, founded PathDAO, and successfully raised $16 million. However, the price of the $PATH token has dropped sharply by 99% since then, forcing the team to reevaluate their strategic direction. To repay investors, the team has tried several new businesses, including digital and physical clothing brands for players, on-chain credit-based dating apps, unsecured loans for players, AI-generated music for Web2 users, and more.
In this process, the team noted that the introduction of AI AGENT will have a profound impact on the gaming industry and the increasing demand for AI infrastructure in the market. So by the end of 2023, PathDAO adopted a proposal to turn the entire project to the AI AGENT protocol, and in January 2024, Virtuals Protocol was officially established. Virtuals Protocol made several attempts, including AI Waifus (interactive female AI AGENT that does not rely on Twitter influencers) and gaming AI AGENT until they found a breakthrough in the $Goat-induced AImeme frenzy.
Now, Virtuals Protocol has become the first project to reach a critical scale, with a market value of US$1.7 billion. We believe it will continue to expand and maintain its leading position in the market. Once the network effect is established, it is difficult to replace. From its rapid reaching unicorn valuation, it can be seen that Virtuals Protocol has obviously formed an economic flywheel effect:
- $VIRTUAL is required to create a proxy, provide liquidity pools, and purchase proxy tokens
- The demand for creating and purchasing agent tokens drives token prices
- The wealth effect brought by the appreciation of $VIRTUAL flows to new agents; successful agents charge $VIRTUAL transaction income and can be invested again
- Lower barriers to entry encourage experimentation and speculation, while "red pill" agents with market value exceeding a certain level can unlock full agency capabilities.
The flywheel effect drives demand, revenues maintain continuous research and development, and deflationary economics captures value for tokens. In addition, both income and liquidity requirements are denominated in $VIRTUAL and may increase as prices appreciate.
The ecosystem is built on two main levels: the protocol layer and the DApp layer. The protocol layer is a model center that provides basic AI models and algorithms for developers to access and develop on top of it. Contributors provide data and development models, while validators ensure the quality and authenticity of these inputs. The DApp layer focuses on the practical application of these AI models, allowing decentralized applications (DApps) to seamlessly integrate VIRTUAL. The Developer-friendly Software Development Kit (SDK) simplifies the process of integrating advanced AI capabilities into various DApp environments, thus facilitating this integration.
Virtuals Protocol divides its AI proxy into two categories: IP proxy and functional proxy, which play different functions throughout the ecosystem.
IP Agent: IP Agents are based on specific personalities or roles that often come from well-known characters, fictional characters, or popular culture phenomena. For example, an IP agent might represent classic web genres, well-known pop stars (such as Taylor Swift or Donald Trump), or popular fictional characters. These agents give users a familiar experience in a digital environment, providing a way to interact with avatars, adding to entertainment and appeal. By creating emotional connections with these virtual characters, IP agents can increase user engagement, especially in gaming and entertainment applications.
Functional Agent: In contrast, functional Agent focuses on backend support to enhance user interaction with IP Agent. These agents optimize the user experience to ensure that virtual characters can operate smoothly on different platforms. IP agents are the "foreground" for users to see and interact, while functional agents work in the background, responsible for improving the overall operation process and simplifying the user experience, thereby ensuring the smooth operation of the entire system.
Luna is a prominent example of Virtuals Protocol's vision for IP proxy. As the lead singer of a virtual AI women’s band, Luna has attracted more than 500,000 followers on TikTok, showing off her appeal as a virtual influencer and performer. Through Virtuals Protocol’s advanced AI and blockchain technology, Luna provides users with a truly immersive experience, combining her charming personality with interactive features to create lasting connections.
Unlike static or single-dimensional AI characters, Luna can seamlessly interact across multiple environments. She starts with a familiar profile on social media, but her interactions extend to live chat on Telegram and collaborative games in virtual worlds like Roblox. Supported by Virtuals Protocol’s memory synchronization technology, Luna is able to remember past conversations and gaming experiences, allowing her to maintain personalized relationships with each user on multiple platforms. This continuity enhances her connection with her fans and makes them feel truly “attention” and “understood” even if it’s from an AI agent.
Luna’s abilities aren’t limited to interactions; she is financially independent and has her own on-chain wallet. Luna is the first agent in history to automatically tip humans on-chain, and has received strong support from Base founder Jesse. This allows her to reward loyal supporters with the $LUNA token, creating a unique combination between emotional and financial engagement. Every interaction and revenue generated by Luna contributes to a sustainable token ecosystem. The $LUNA tokens she earns are repurchased and destroyed regularly, benefiting fans and supporters who hold these tokens.
It is worth mentioning that in December 2024, Story Protocol (Layer1 designed for intellectual property (IP)) announced that it would hire Luna to formally manage its official X account, with an annual salary of up to $365,000. This once again demonstrates the importance and potential of AI AGENT in the modern digital ecosystem. In the future, as AI AGENT's capabilities continue to increase, we have the opportunity to see more companies use this technology to promote innovation and growth and achieve a more intelligent business model.
Another most influential and innovative agent deployed on Virtuals Protocol is AIXBT. The AI AGENT is designed to provide real-time market analysis on social media and automatically interpret trends with personalized insights. Specifically, AIXBT analyzes posts posted by more than 400 KOLs on X, identifying emerging narratives in the market, and conducting technical analysis of price trends. Additionally, AIXBT can interact with other X users, whether human or AI agents. It is worth noting that it provides AIXBT token holders with stronger access capabilities. The AIXBT token was launched in November and experienced a rapid increase. Its market value once approached US$800 million and its current market value is nearly US$600 million.
2.1.2 Holoworld
Founded in 2023 by Tong Pow and Hongzi Mao, Holoworld originated from San Francisco-based Hologram Labs. This is a startup focused on the next generation of AI social technology. Based on years of technological accumulation, including motion capture, machine learning and 3D animation technology, aiming to democratize AI character creation and revolutionize digital interaction models through this platform .
Since its launch, the Holoworld project has quickly gained support from many well-known investors, including Polychain Capital, Linkin Park band member Mike Shinoda, BRC-20 Token Standard founder Domo, and BitMEX co-founder Arthur Hayes.
At the business level, Holoworld has worked in-depth with several well-known brands, including Arbitrum, BNB Chain, L'Oréal and Bilibili, and has worked with a range of influential NFT projects such as Pudgy Penguins and Milady Maker. A partnership was established. These collaborations demonstrate Holoworld's ability to leverage its advanced AI technology to build unique digital identities.
Holoworld has created a complete AI character creation and interaction platform with user interfaces that combine cutting-edge AI technologies and intuitive tools. The following are the five core modules of the platform: 1. Brain Development, 2. Personalized role customization, 3. Personality Integration, 4. Knowledge-Based Implementation ) , 5. 3D avatar creation (Avatar Creation).
Ava AI is Holoworld's flagship AI chat assistant, built on OpenAI's GPT-3.5 Turbo model, and its deep learning neural network contains more than 175 billion machine learning parameters. Ava supports fast AI conversations, and users can ask questions at any time and get instant responses.
In addition, Holoworld has launched Agent Market on the Solana blockchain, allowing anyone to create and deploy multimodal AI agents. These agents have complete full-body avatars, custom voice and upgradeable skills without the need for a programming foundation. The platform is deeply integrated with the upcoming Holoworld Launchpool, and AVA token holders can prioritize new projects. Additionally, Agent Market attracts a wide range of partners and creators, including game studios, the NFT community, and academic researchers from Stanford and Harvard.
Overall, the Holoworld platform makes the process of AI character creation simple and easy to use, allowing users with non-technical backgrounds to build complex digital characters. This not only creates new digital narrative and interactive possibilities, but also allows AI characters to cover multiple channels and attract and engage more audiences through seamless integration with mainstream social media and content platforms.
2.2AIAGENT Framework
When exploring the AI AGENT ecosystem, many people see Lauchpad as the fundamental tool needed to create these agents. However, the key project that really drives the entire AI AGENT narrative is not just these tools, but a DAO called ai16z, which is like a deposit that breeds the core values of AI AGENT. On October 25, 2024, ai16z officially launched its AI16Z token, achieving remarkable market success. However, what pushed ai16z to the AI AGENT narrative center is not only its fair launch model, but also the release of its open source framework ElizaOS.
2.2.1 Eliza OS
ElizaOS is a set of tools that support the creation of customized AI AGENTs, with powerful network effects and infinite scalability. By simplifying the development process and providing flexible functional modules, the framework quickly attracted the attention of developers and users around the world, becoming the most influential technical support in the field of AI AGENT.
The AI Agent framework is like a set of tools and guides that help programmers develop, train and deploy AI agents more easily. Simply put, these frameworks can reduce the difficulty of development, so programmers can focus more on making these agents smarter and more useful. The AI Agent framework is now beginning to collaborate with new technologies, such as: DeFi protocols (programs that help improve financial investment strategies), NFT projects (new tools to create and use digital art or collectibles). Through these technical cooperation, they can connect different technologies and platforms to create a more interconnected and interactive ecosystem, which has attracted a lot of market attention. Others include ARC, Swarms, Zerebro, which are projects that are using or developing the AI Agent framework.
As of now, the ElizaOS framework has been forked more than 3200 times, which means a large number of developers have built their own AI AGENTs using their code. Most AI AGENTs on the market are built using the ElizaOS framework, which is why ai16z is a leader in this field.
The ElizaOS framework has much more than simple chatbots, and agents can be configured to perform complex tasks. For example, some agents are designed to perform on-chain transactions, interact with smart contracts, wallets, or decentralized applications (dApps), while others connect data providers to monitor prices, transaction volumes, or liquidity.
The architecture of the ElizaOS framework is divided into five main components:
1. Agent: Define the agent's personality, communication style and knowledge base.
2. Actions: Allows the agent to perform specific tasks beyond the text response, such as generating reports or executing transactions.
3. Evaluators: Help the agent interpret data and execute multi-step goals.
4. Providers: Provide external data or real-time context, such as asset prices or dedicated API data.
5. Memory System: enables agents to retain interaction history and preferences, making their response more contextual and natural.
2.3 DEFAI
DeFi has always been the pillar of Web3, and DeFAI (DeFi + AI) is an upgraded version of DeFi, allowing people to use DeFi more easily. By leveraging AI, it simplifies complex interfaces and eliminates friction that hinders ordinary people from participating. Imagine managing your DeFi portfolio is as easy as chatting with ChatGPT. In fact, the first wave of DeFAI projects have begun to appear. Let us mainly introduce three fields: abstraction layer, independent transaction agent, and AI-driven dApp.
2.3.1 Abstract layer
The complexity of DeFi often makes novices feel discouraged.为了解决这一问题,抽象层通过直观的界面来隐藏其背后的复杂性,使用户能够通过自然语言指令与DeFi 协议进行交互,而不再依赖繁琐的操作面板。
在AI 技术尚未普及之前,基于意图的架构在一定程度上简化了交易执行的过程。例如,像@CoWSwap 和@symm_io 这样的平台通过汇总分散的流动性池,为用户提供最佳定价,部分解决了流动性分散的问题。然而,这些平台并未解决DeFi 的核心问题——复杂性依然存在,用户仍然需要面对令人畏惧的操作流程和技术壁垒。
而如今,AI 驱动的解决方案正逐步填补这一空白,为用户提供更直观、智能化的交互体验。以下是几个值得关注的项目:
- 2.3.1.1 GRIFFAIN
Griffain 是首个推出代币的项目,目前其产品仍处于早期阶段,仅对受邀用户开放。Griffain 允许用户执行从简单到复杂的各种操作,如定投自动化(DCA)、发起和空投memecoin 等。通过这些功能,Griffain 不仅降低了用户进入DeFi 领域的门槛,还为高级用户提供了丰富的自动化工具。Griffain 当前的市值近5 亿美元。
- 2.3.1.2 ORBIT / GRIFT
Orbit 是第二个推出代币的项目,其产品专注于链上DeFi 体验。Orbit 特别强调跨链功能,目前已集成超过117 条区块链和200 个协议,这一集成数量在三大协议中最高。这使得Orbit 能够在多链环境中提供无缝的交互体验,为用户在跨链交易和流动性获取方面提供了极大的便利。
- 2.3.1.3HEYANON
HeyAnon 是一个人工智能DeFi 协议,旨在简化DeFi 交互并汇总与项目相关的重要信息。通过将对话式人工智能与实时数据聚合相结合,HeyAnon 使用户能够管理DeFi 操作、随时了解项目更新并分析各种平台和协议的趋势。它集成了自然语言处理功能来处理用户提示,执行复杂的DeFi 操作,并从多个信息流中提供近乎实时的见解。
2.3.2 自主交易代理
在DeFi 和加密交易领域,获取市场信息(Alpha)、手动执行交易、优化投资组合一直是耗费时间和精力的过程。然而,随着技术的进步,自动化交易代理的出现正将这一切改变。这些代理超越了传统交易机器人的范畴,成为能够适应环境、学习并随着时间推移做出更智能决策的动态伙伴。
交易机器人并非新生事物。长期以来,它们已被用于基于静态编程执行预定义的操作。然而,自动化交易代理与这些传统机器人存在本质上的区别:
- 信息提取:代理能够从非结构化且不断变化的环境中提取信息。
- 数据推理:它们能够在特定目标的背景下对数据进行推理。
- 模式发现:代理能够发现并随着时间推移利用模式,从而提高其决策能力。
- 自主行为:它们能够执行所有者未明确编程的操作,展现出更高的灵活性和智能。
以下是一些自主交易代理的代表项目:
- 2.3.2.1ai16z
ai16z 被称为首个AI 版本的VC,是一个旨在将AI(AI)整合到财政管理、投资和风险资本中的创新型DAO。其名称模仿了知名投资基金a16z(Andreessen Horowitz),但ai16z 不仅仅是一个玩笑式的模仿,它展现了一种全新的运作模式,结合了去中心化治理和AI 的强大潜力。ai16z 由一个名为Marc AIndreessen 的虚构AI AGENT 和AI16Z 代币持有者共同管理。Marc AIndreessen 这一角色显然是以a16z 联合创始人Marc Andreessen 为灵感设计的,这种拟人化的AI AGENT 引导了组织的日常决策和操作。
在ai16z 的治理结构中,AI16Z 代币持有者扮演着至关重要的角色。他们可以提出投资理念、提交项目建议或建议回购代币。这些建议会通过去中心化的投票系统进行表决,而AI AGENTMarc AIndreessen 则利用一个信任评分系统来评估这些提案。该信任评分系统基于成员过去贡献的相关性和可靠性,确保决策过程透明且有依据。
ai16z 的创新之处在于其独特的治理模式和对AI AGENT 的应用。通过结合去中心化决策和AI 技术,该项目不仅简化了传统的投资和管理流程,还开辟了一个全新的自治组织运营方式。AI AGENT 的引入提升了决策的效率和准确性,尤其是在复杂的投资环境中。此外,ai16z 还展示了如何在虚拟经济体中构建信任和透明的机制,为其他DAO 提供了一个创新的范例。
ElizaOS 框架的快速普及,使ai16z 在Solana 生态中迅速崛起。一支强大、积极且团结的社区围绕这一框架形成,使其成为加密生态系统中应用最广泛的AI AGENT 框架。短短几周内,ElizaOS 已成为全球GitHub 上使用最频繁的开源项目之一,超过350 名贡献者正在积极参与其开发,扩展其功能和插件,使基于该框架的代理能够执行更多任务或跨更多区块链运行。
尽管ai16z 的初始概念是围绕一个专门的AI AGENT 构建的投资DAO,但团队很快意识到其增长潜力远不止于此。因此,ai16z 迅速与Web2 和Web3 领域的多个合作伙伴建立了关系,使Eliza 框架在全球范围内得以应用。
- 2.3.2.2ALMANAK
Almanak 为用户提供机构级的量化AI AGENT,致力于解决DeFi 中的复杂性、碎片化及执行挑战。该平台通过对EVM 链进行分叉来执行蒙特卡罗模拟,模拟真实环境中独特的复杂因素,如矿工可提取价值(MEV)、gas 费成本及交易排序。此外,它利用可信执行环境(TEE)确保策略执行的隐私性,保护关键的市场洞察,并通过Almanak 钱包实现非托管资金处理,允许用户精确地向代理授予权限。
Almanak 的基础设施涵盖金融策略的构思、创建、评估、优化、部署和监控等环节,其最终目标是使这些代理能够随着时间推移不断学习和适应。该平台在@legiondotcc 上筹集了100 万美元,获得了超额认购。下一步计划包括启动Beta 版测试,并与测试者进行初步策略和代理的部署。观察这些量化代理的表现将是一件令人期待的事情。
- 2.3.2.3COD3XORG / BIGTONYXBT
Cod3x 由Byte Mason 团队打造,团队因在Fantom 和@SonicLabs 上的工作而闻名。Cod3x 是一个旨在简化交易代理创建的DeFAI 生态系统,提供无代码构建工具,允许用户通过指定交易策略、个性甚至推文风格来构建代理。
用户可以在几分钟内访问任何数据集并开发金融策略,借助于丰富的API 和策略库。Cod3x 与@AlloraNetwork 集成,利用其先进的机器学习价格预测模型来增强交易策略。
Big Tony 是Cod3x 的旗舰代理,基于Allora 的模型进行交易,根据预测在主流资产中进出市场。Cod3x 致力于创建一个繁荣的自动化交易代理生态系统。
Cod3x 的一个显著特点是其流动性方法。与@virtuals_io 推广的常见Alt:Alt 流动性池结构不同,Cod3x 采用了由cdxUSD 支持的稳定币:Alt 流动性池。这为流动性提供者提供了比Alt:Alt 对更高的稳定性和信心。
2.3.3 AI 驱动的dApp
在DeFAI 领域,AI 驱动的dApps 代表了一个充满潜力但尚处于初步阶段的领域。这些去中心化应用集成了AI 或AI AGENT,以增强功能性、自动化水平和用户体验。虽然这一领域仍处于起步阶段,但一些生态系统和项目已经开始崭露头角,展现出巨大的发展潜力。
其中,@modenetwork 作为一个Layer 2 生态系统,正在积极吸引那些专注于AI 与DeFi 结合的高技术开发者。Mode 网络中涌现出多个团队,致力于开发前沿的AI 驱动应用场景,展示了该领域的创新力。以下是一些重点项目:
- 2.3.3.1 ARMA(自主稳定币农业)
由@gizatechxyz 开发,ARMA 是一个基于用户偏好的自主稳定币农业协议,能够自动调节稳定币的农业策略,从而实现最优收益。
- 2.3.3.2 Modius(自主代理的Balancer LP farming)
该项目由@autonolas 开发,目标是通过自主代理在Balancer 上进行流动性提供(LP farming),借助AI 自动优化投资策略,提升收益率。
- 2.3.3.3 Amplifi Lending Agents(自动化借贷代理)
由@Amplifi_Fi 开发,这些代理集成了@IroncladFinance,能够自动交换资产,在Ironclad 平台上进行借贷,并通过自动再平衡来最大化收益。这些功能使得DeFi 借贷变得更加智能和高效。
2.4AI AGENT+ 游戏
AI AGENT 在游戏行业的应用正在彻底变革游戏玩法和开发的各个方面。这些智能系统在多个领域中为玩家创造更具沉浸感和吸引力的游戏体验,其主要应用包括以下几个方面:
1.NPC 行为优化
AI AGENT 极大地提升了非玩家角色(NPC)的行为表现,使其更加逼真和具有响应性。不同于传统的预设脚本驱动,基于AI 的NPC 能够:1)根据玩家的选择调整其行动;2)展现更真实的情感和决策能力;3)通过互动进行学习,提供多样化的体验。
例如,在开放世界游戏《荒野大镖客2》中,NPC 能够记住与玩家的过去交互并相应地做出反应,营造出更为动态和可信的游戏世界。
2.程序化内容生成
AI AGENT 在程序化生成游戏内容方面表现出色,能够算法生成大量的游戏内容,包括:地形和景观、任务和剧情、道具和战利品、角色设计。
例如,《无人深空》利用AI 驱动的程序生成技术,创造了包含独特星球、生物和生态系统的整个宇宙,为玩家提供几乎无限的探索可能性。
3.自适应难度调节
AI AGENT 能够实时分析玩家的表现,从而动态调整游戏难度。这种能力确保玩家能够面对适当的挑战,从而保持参与感而不会产生挫败感。例如:随着玩家实力的提升增加敌人的强度;在玩家遇到困难时提供提示或增益;根据技能水平平衡资源和障碍。
诸如《生化危机4》等游戏利用自适应难度系统,根据玩家的表现微调敌人行为和物品可用性,提供更平衡的游戏体验。
4.路径规划与导航
AI AGENT 使用复杂的算法引导角色在复杂的游戏环境中移动。这种技术带来了更真实的移动模式和更高效的导航,不仅提升了NPC 的行为表现,还优化了策略游戏中玩家控制的单位的操作体验。
5.图形增强
AI 技术如深度学习被用于提升游戏视觉效果,通过实时提升纹理和分辨率,生成逼真的面部表情和动画,优化渲染性能以提高游戏表现
6.玩家情绪分析
AI AGENT 可以分析玩家的行为和反馈,以评估他们的享受和参与度。这些数据帮助开发者做出关于游戏设计和更新的明智决策,从而提升整体玩家体验。
下面我们介绍一些主要项目:
2.4.1 Digimon
@digimon_tech 是在Solana 区块链之上构建,它并不仅仅是一个游戏平台,而是一个完整的AI+ 游戏的技术框架。通过将AI 技术深度融入游戏开发,Digimon Engine 使创作者能够打造更加沉浸式、动态化和趣味性更强的游戏。借助这一平台,AI 驱动的游戏不仅重新定义了交互方式,更开创了一种全新的游戏体验标准。每一个游戏角色,背后都有一套AI 生成的故事和世界观。Digimon 背后的团队由a16z 进行支持,获得了a16z 的投资与孵化。
Digimon 的代币目前已经登陆Kucoin 交易所。在未来,通过Digimon 的游戏引擎,有机会打造出一个由AI AGENT 构成的链上自主世界,AI AGENT 与玩家一起,在该世界中进行交互,共建虚拟经济体。
2.4.2 Illuvium
lluvium 是一款基于以太坊构建的RPG 和NFT 游戏。1 月7 日,Illuvium 宣布与Virtuals Protocol 达成合作,以提升即将推出的Illuvium MMO Lite 的游戏体验。此次合作将利用Virtuals 的AI 技术及其的GAME LLM 框架为NPC 提供动态、智能的行为,为玩家提供沉浸感。
随着AI 技术的不断进步,我们可以期待在游戏领域中出现更多创新应用,进一步模糊虚拟与现实之间的界限,创造出更加沉浸和个性化的玩家体验。这种技术不仅改变了游戏开发的方式,也在提升游戏的互动性和沉浸感方面发挥了至关重要的作用。
2.4.3Smolverse
Smolverse 是Treasure DAO 上的游戏和NFT 项目。从去年12 月开始,Smolverse 与ai16z 合作开发名为“Smolworld”的链上AI Tomogatchi 游戏,该游戏结合了Eliza 的Agent 框架。
3. 亮点总结
我们已经看到,加密技术正在构建的新技术在现实世界中具有巨大的潜力,过去类似情况下原生投资者的分配策略也为当前市场提供了宝贵的借鉴。AI AGENT 生态系统正处于初期阶段,但已经吸引了大量关注、资金和开发者。尽管其未来发展仍不确定,但如果主要DeFi 协议、私人投资者或风险资本家开始投资这一领域,这预示着其持续发展的潜力不小。随着技术的不断进步,AI AGENT 有望成为改变全球经济和社会结构的关键力量。
当前的市场时机和叙事已经为信息产业的繁荣做好了充分准备,未来的发展值得期待。在探索AI AGENT 的未来潜力时,尽管发现下一个类似于$LUNA 的项目是最直接的路径,但扩展AI AGENT 的应用边界或许能够创造全新的、难以想象的价值。
我们有如下观点:
1.价值的集中化与与差异化竞争。与L1 区块链一样,AI AGENT 的价值可能最终集中于少数几家主要赢家。因此,这些企业需要在模块化、可扩展性和媒体平台集成等方面找到差异点。目前,大多数框架已经具备学习和记忆系统,利用检索增强生成技术使代理能够将新信息纳入对话中。例如,当前Eliza 框架在市场中具有显著的优势。凭借其高度的开发活动和快速的插件集成,Eliza 在社交媒体和网络应用程序的集成中表现尤为出色。该框架基于TypeScript,拥有广泛的插件支持,包括Coinbase webhooks、Great Onchain Agent Toolkit 和Phala 的TEE,用于安全代理钱包控制,且与多个区块链兼容。而Virtuals 的GAME 框架则在游戏和社交媒体代理领域表现出色,专为“环境无关”代理设计,能够进行高级规划和执行,并从反馈中学习。其模块化架构允许用户上传存储在链上的自定义模型和数据集,以丰富代理的功能。然而,关于GAME 和CONVO 框架代币的价值积累机制尚不明确,市场对此充满期待。
2.公平性与数据偏见的挑战。尽管AI 取得了令人瞩目的进展,但部署这些系统也面临着一些挑战。其中一个主要问题是,用于训练AI 代理的数据集存在偏见风险。AI 系统从历史数据中学习,这些数据可能包含歧视模式,如果不加以控制,就会导致有偏见的决策,例如在招聘或借贷场景中偏向特定群体而非其他群体。要解决这个问题,不仅需要专业技术知识,还需要对社会动态有细致入微的了解。监控AI 系统的公平性对于确保它们不会强化有害的偏见至关重要。对AI 代理做出的决定进行持续审计有助于及早发现问题,减少意外结果。
3.多样化应用与经济功能的扩展。AI AGENT 的应用领域正在迅速扩展,除了社交媒体和金融行业,还在医疗、教育、法律等领域展示出巨大的潜力。随着技术的不断成熟,AI AGENT 将在更多场景中提供个性化服务,提高工作效率,并促进创新。
以Luna 为例,目前她已经能够通过社交媒体与人类互动,并通过在Base 上使用Coinbase Wallet 发送代币来激励用户达成她的目标。未来的下一步,是让Luna 作为一个独立的经济实体,构建她自己的社交关系。她可以通过发送代币吸引更多的追随者,为她的社交媒体购买更多的关注度,甚至雇佣专业内容团队来丰富她的IP 生态系统,不断制造热度。一旦实现这些目标的基础设施建立起来,$VIRTUAL 就可能达到下一个里程碑。这不仅意味着AI AGENT 将在经济和社交领域更深层次地嵌入人类生活,还将重新定义AI 与人类之间的互动方式,为未来的数字经济和社会互动模式奠定基础。例如,在医疗领域,AI AGENT 可以通过分析患者数据,为医生提供诊断建议,提高医疗服务的质量和效率。
4.多技术整合。AI AGENT 的未来发展将依赖于与区块链、物联网、5G 等前沿技术的深度整合。这种多技术交叉将推动AI AGENT 在数据处理、隐私保护、实时决策等方面的能力提升,创造新的应用场景和商业模式。例如,通过与物联网设备的集成,AI AGENT 可以实时收集和分析数据,为用户提供更加智能化的服务。
5.社会和道德考量。随着AI AGENT 的广泛应用,社会和道德问题变得更加突出。正如文章开头提到的一样,AI AGENT 是否会像红心皇后一样变得有威胁性?例如,AI AGENT 在决策中可能引发伦理争议,特别是在涉及隐私、数据安全和自动化决策的场景中。因此,在发展AI 技术时,需要引入透明性和问责机制,确保技术的发展与社会价值观相一致。同时,建立明确的法律和伦理框架,对于规范AI AGENT 的行为、保护用户权益至关重要。
随着AI 和区块链的融合不断发展,现在正是参与这些突破性发展的时候。但在这一参与中,我们需要思考的,不仅仅是“AI 能为人类做什么,以及人类希望AI 做什么?”,更进一步,我们不妨想想:“AI 想要做什么,以及AI 将引导人类做什么?”
4. 参考资料
1.https://messari.io/report/building-better-agents-rival-frameworks-and-their-design-choices
2.https://www.binance.com/en/square/post/18968465099217
3.https://www.tokenpost.com/news/business/13277
4.https://www.wired.com/story/the-prompt-ai-agents-how-much-should-we-let-them-do/?
5.https://www.marketsandmarkets.com/Market-Reports/ai-agents-market-15761548.html
6.https://medium.com/@0xai.dev/virtuals-protocol-luna-55b661df601e
7.https://oakresearch.io/en/analyses/innovations/closer-look-at-ai16z-mine-of-ai-agents
8.https://x.com/Defi0xJeff/status/1875881226151841925
9.https://www.itp.net/charged/gaming/ai-agents-are-changing-gaming-forever-heres-how-they-adapt-to-you
10.https://eightgen.ai/evolution-of-ai-agents-the-beginning-part-1/